parent
e4a3659fdb
commit
09838bd6f9
@ -1,124 +0,0 @@
|
||||
# `Idea2Image` Documentation
|
||||
|
||||
## Table of Contents
|
||||
|
||||
1. [Introduction](#introduction)
|
||||
2. [Idea2Image Class](#idea2image-class)
|
||||
- [Initialization Parameters](#initialization-parameters)
|
||||
3. [Methods and Usage](#methods-and-usage)
|
||||
- [llm_prompt Method](#llm-prompt-method)
|
||||
- [generate_image Method](#generate-image-method)
|
||||
4. [Examples](#examples)
|
||||
- [Example 1: Generating an Image](#example-1-generating-an-image)
|
||||
5. [Additional Information](#additional-information)
|
||||
6. [References and Resources](#references-and-resources)
|
||||
|
||||
---
|
||||
|
||||
## 1. Introduction <a name="introduction"></a>
|
||||
|
||||
Welcome to the documentation for the Swarms library, with a focus on the `Idea2Image` class. This comprehensive guide provides in-depth information about the Swarms library and its core components. Before we dive into the details, it's crucial to understand the purpose and significance of this library.
|
||||
|
||||
### 1.1 Purpose
|
||||
|
||||
The Swarms library aims to simplify interactions with AI models for generating images from text prompts. The `Idea2Image` class is designed to generate images from textual descriptions using the DALLE-3 model and the OpenAI GPT-4 language model.
|
||||
|
||||
### 1.2 Key Features
|
||||
|
||||
- **Image Generation:** Swarms allows you to generate images based on natural language prompts, providing a bridge between textual descriptions and visual content.
|
||||
|
||||
- **Integration with DALLE-3:** The `Idea2Image` class leverages the power of DALLE-3 to create images that match the given textual descriptions.
|
||||
|
||||
- **Language Model Integration:** The class integrates with OpenAI's GPT-3 for prompt refinement, enhancing the specificity of image generation.
|
||||
|
||||
---
|
||||
|
||||
## 2. Idea2Image Class <a name="idea2image-class"></a>
|
||||
|
||||
The `Idea2Image` class is a fundamental module in the Swarms library, enabling the generation of images from text prompts.
|
||||
|
||||
### 2.1 Initialization Parameters <a name="initialization-parameters"></a>
|
||||
|
||||
Here are the initialization parameters for the `Idea2Image` class:
|
||||
|
||||
- `image` (str): Text prompt for the image to generate.
|
||||
|
||||
- `openai_api_key` (str): OpenAI API key. This key is used for prompt refinement with GPT-3. If not provided, the class will attempt to use the `OPENAI_API_KEY` environment variable.
|
||||
|
||||
- `cookie` (str): Cookie value for DALLE-3. This cookie is used to interact with the DALLE-3 API. If not provided, the class will attempt to use the `BING_COOKIE` environment variable.
|
||||
|
||||
- `output_folder` (str): Folder to save the generated images. The default folder is "images/".
|
||||
|
||||
### 2.2 Methods <a name="methods-and-usage"></a>
|
||||
|
||||
The `Idea2Image` class provides the following methods:
|
||||
|
||||
- `llm_prompt()`: Returns a prompt for refining the image generation. This method helps improve the specificity of the image generation prompt.
|
||||
|
||||
- `generate_image()`: Generates and downloads the image based on the prompt. It refines the prompt, opens the website with the query, retrieves image URLs, and downloads the images to the specified folder.
|
||||
|
||||
---
|
||||
|
||||
## 3. Methods and Usage <a name="methods-and-usage"></a>
|
||||
|
||||
Let's explore the methods provided by the `Idea2Image` class and how to use them effectively.
|
||||
|
||||
### 3.1 `llm_prompt` Method <a name="llm-prompt-method"></a>
|
||||
|
||||
The `llm_prompt` method returns a refined prompt for generating the image. It's a critical step in improving the specificity and accuracy of the image generation process. The method provides a guide for refining the prompt, helping users describe the desired image more precisely.
|
||||
|
||||
### 3.2 `generate_image` Method <a name="generate-image-method"></a>
|
||||
|
||||
The `generate_image` method combines the previous methods to execute the whole process of generating and downloading images based on the provided prompt. It's a convenient way to automate the image generation process.
|
||||
|
||||
---
|
||||
|
||||
## 4. Examples <a name="examples"></a>
|
||||
|
||||
Let's dive into practical examples to demonstrate the usage of the `Idea2Image` class.
|
||||
|
||||
### 4.1 Example 1: Generating an Image <a name="example-1-generating-an-image"></a>
|
||||
|
||||
In this example, we create an instance of the `Idea2Image` class and use it to generate an image based on a text prompt:
|
||||
|
||||
```python
|
||||
from swarms.agents import Idea2Image
|
||||
|
||||
# Create an instance of the Idea2Image class with your prompt and API keys
|
||||
idea2image = Idea2Image(
|
||||
image="Fish hivemind swarm in light blue avatar anime in zen garden pond concept art anime art, happy fish, anime scenery",
|
||||
openai_api_key="your_openai_api_key_here",
|
||||
cookie="your_cookie_value_here",
|
||||
)
|
||||
|
||||
# Generate and download the image
|
||||
idea2image.generate_image()
|
||||
```
|
||||
|
||||
---
|
||||
|
||||
## 5. Additional Information <a name="additional-information"></a>
|
||||
|
||||
Here are some additional tips and information for using the Swarms library and the `Idea2Image` class effectively:
|
||||
|
||||
- Refining the prompt is a crucial step to influence the style, composition, and mood of the generated image. Follow the provided guide in the `llm_prompt` method to create precise prompts.
|
||||
|
||||
- Experiment with different prompts, variations, and editing techniques to create unique and interesting images.
|
||||
|
||||
- You can combine separate DALLE-3 outputs into panoramas and murals by careful positioning and editing.
|
||||
|
||||
- Consider sharing your creations and exploring resources in communities like Reddit r/dalle2 for inspiration and tools.
|
||||
|
||||
- The `output_folder` parameter allows you to specify the folder where generated images will be saved. Ensure that you have the necessary permissions to write to that folder.
|
||||
|
||||
---
|
||||
|
||||
## 6. References and Resources <a name="references-and-resources"></a>
|
||||
|
||||
For further information and resources related to the Swarms library and DALLE-3:
|
||||
|
||||
- [DALLE-3 Unofficial API Documentation](https://www.bing.com/images/create): The official documentation for the DALLE-3 Unofficial API, where you can explore additional features and capabilities.
|
||||
|
||||
- [OpenAI GPT-3 Documentation](https://beta.openai.com/docs/): The documentation for OpenAI's GPT-3, which is used for prompt refinement.
|
||||
|
||||
This concludes the documentation for the Swarms library and the `Idea2Image` class. You now have a comprehensive guide on how to generate images from text prompts using DALLE-3 and GPT-3 with Swarms.
|
@ -1,94 +0,0 @@
|
||||
# `OmniModalAgent` Documentation
|
||||
|
||||
## Overview & Architectural Analysis
|
||||
The `OmniModalAgent` class is at the core of an architecture designed to facilitate dynamic interactions using various tools, through a seamless integration of planning, task execution, and response generation mechanisms. It encompasses multiple modalities including natural language processing, image processing, and more, aiming to provide comprehensive and intelligent responses.
|
||||
|
||||
### Architectural Components:
|
||||
1. **LLM (Language Model)**: It acts as the foundation, underpinning the understanding and generation of language-based interactions.
|
||||
2. **Chat Planner**: This component drafts a blueprint for the steps necessary based on the user's input.
|
||||
3. **Task Executor**: As the name suggests, it's responsible for executing the formulated tasks.
|
||||
4. **Tools**: A collection of tools and utilities used to process different types of tasks. They span across areas like image captioning, translation, and more.
|
||||
|
||||
|
||||
## Structure & Organization
|
||||
|
||||
### Table of Contents:
|
||||
1. Class Introduction and Architecture
|
||||
2. Constructor (`__init__`)
|
||||
3. Core Methods
|
||||
- `run`
|
||||
- `chat`
|
||||
- `_stream_response`
|
||||
4. Example Usage
|
||||
5. Error Messages & Exception Handling
|
||||
6. Summary & Further Reading
|
||||
|
||||
### Constructor (`__init__`):
|
||||
The agent is initialized with a language model (`llm`). During initialization, the agent loads a myriad of tools to facilitate a broad spectrum of tasks, from document querying to image transformations.
|
||||
|
||||
### Core Methods:
|
||||
#### 1. `run(self, input: str) -> str`:
|
||||
Executes the OmniAgent. The agent plans its actions based on the user's input, executes those actions, and then uses a response generator to construct its reply.
|
||||
|
||||
#### 2. `chat(self, msg: str, streaming: bool) -> str`:
|
||||
Facilitates an interactive chat with the agent. It processes user messages, handles exceptions, and returns a response, either in streaming format or as a whole string.
|
||||
|
||||
#### 3. `_stream_response(self, response: str)`:
|
||||
For streaming mode, this function yields the response token by token, ensuring a smooth output agent.
|
||||
|
||||
## Examples & Use Cases
|
||||
Initialize the `OmniModalAgent` and communicate with it:
|
||||
```python
|
||||
import os
|
||||
|
||||
from dotenv import load_dotenv
|
||||
|
||||
from swarms.agents.omni_modal_agent import OmniModalAgent, OpenAIChat
|
||||
from swarms.models import OpenAIChat
|
||||
|
||||
# Load the environment variables
|
||||
load_dotenv()
|
||||
|
||||
# Get the API key from the environment
|
||||
api_key = os.environ.get("OPENAI_API_KEY")
|
||||
|
||||
# Initialize the language model
|
||||
llm = OpenAIChat(
|
||||
temperature=0.5,
|
||||
model_name="gpt-4",
|
||||
openai_api_key=api_key,
|
||||
)
|
||||
|
||||
|
||||
agent = OmniModalAgent(llm)
|
||||
response = agent.run("Translate 'Hello' to French.")
|
||||
print(response)
|
||||
```
|
||||
|
||||
For a chat-based interaction:
|
||||
```python
|
||||
agent = OmniModalAgent(llm_instance)
|
||||
print(agent.chat("How are you doing today?"))
|
||||
```
|
||||
|
||||
## Error Messages & Exception Handling
|
||||
The `chat` method in `OmniModalAgent` incorporates exception handling. When an error arises during message processing, it returns a formatted error message detailing the exception. This approach ensures that users receive informative feedback in case of unexpected situations.
|
||||
|
||||
For example, if there's an internal processing error, the chat function would return:
|
||||
```
|
||||
Error processing message: [Specific error details]
|
||||
```
|
||||
|
||||
## Summary
|
||||
`OmniModalAgent` epitomizes the fusion of various AI tools, planners, and executors into one cohesive unit, providing a comprehensive interface for diverse tasks and modalities. The versatility and robustness of this agent make it indispensable for applications desiring to bridge multiple AI functionalities in a unified manner.
|
||||
|
||||
For more extensive documentation, API references, and advanced use-cases, users are advised to refer to the primary documentation repository associated with the parent project. Regular updates, community feedback, and patches can also be found there.
|
||||
|
||||
|
||||
|
||||
|
||||
|
||||
|
||||
|
||||
|
||||
|
@ -1,79 +0,0 @@
|
||||
# Module/Class Name: OmniModalAgent
|
||||
|
||||
The `OmniModalAgent` class is a module that operates based on the Language Model (LLM) aka Language Understanding Model, Plans, Tasks, and Tools. It is designed to be a multi-modal chatbot which uses various AI-based capabilities for fulfilling user requests.
|
||||
|
||||
It has the following architecture:
|
||||
1. Language Model (LLM).
|
||||
2. Chat Planner - Plans
|
||||
3. Task Executor - Tasks
|
||||
4. Tools - Tools
|
||||
|
||||
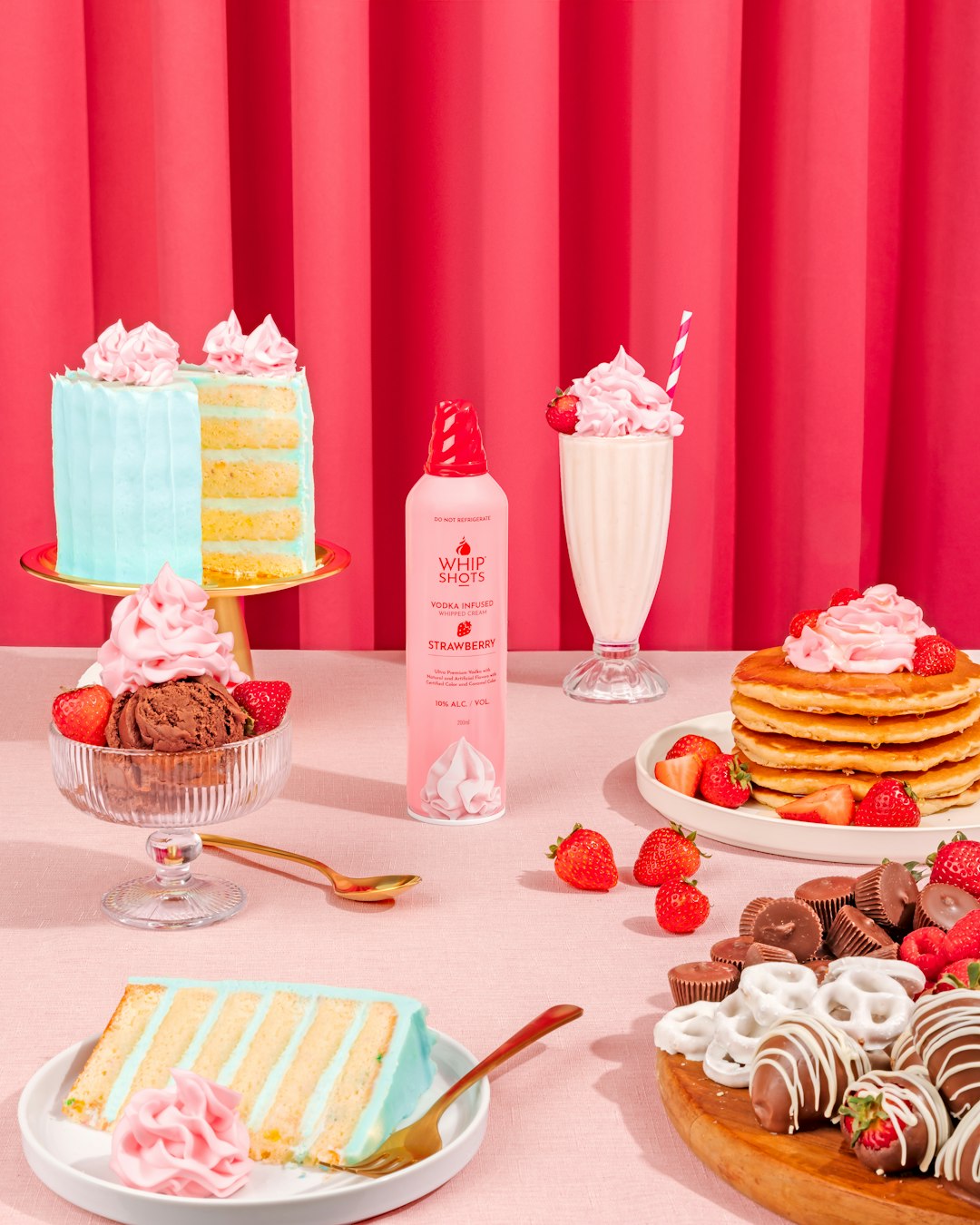
|
||||
|
||||
---
|
||||
|
||||
### Usage
|
||||
from swarms import OmniModalAgent, OpenAIChat
|
||||
|
||||
llm = OpenAIChat()
|
||||
agent = OmniModalAgent(llm)
|
||||
response = agent.run("Hello, how are you? Create an image of how your are doing!")
|
||||
|
||||
---
|
||||
|
||||
---
|
||||
|
||||
### Initialization
|
||||
|
||||
The constructor of `OmniModalAgent` class takes two main parameters:
|
||||
- `llm`: A `BaseLanguageModel` that represents the language model
|
||||
- `tools`: A List of `BaseTool` instances that are used by the agent for fulfilling different requests.
|
||||
|
||||
```python
|
||||
def __init__(
|
||||
self,
|
||||
llm: BaseLanguageModel,
|
||||
# tools: List[BaseTool]
|
||||
):
|
||||
```
|
||||
|
||||
---
|
||||
|
||||
### Methods
|
||||
|
||||
The class has two main methods:
|
||||
1. `run`: This method takes an input string and executes various plans and tasks using the provided tools. Ultimately, it generates a response based on the user's input and returns it.
|
||||
- Parameters:
|
||||
- `input`: A string representing the user's input text.
|
||||
- Returns:
|
||||
- A string representing the response.
|
||||
|
||||
Usage:
|
||||
```python
|
||||
response = agent.run("Hello, how are you? Create an image of how your are doing!")
|
||||
```
|
||||
|
||||
2. `chat`: This method is used to simulate a chat dialog with the agent. It can take user's messages and return the response (or stream the response word-by-word if required).
|
||||
- Parameters:
|
||||
- `msg` (optional): A string representing the message to send to the agent.
|
||||
- `streaming` (optional): A boolean specifying whether to stream the response.
|
||||
- Returns:
|
||||
- A string representing the response from the agent.
|
||||
|
||||
Usage:
|
||||
```python
|
||||
response = agent.chat("Hello")
|
||||
```
|
||||
|
||||
---
|
||||
|
||||
### Streaming Response
|
||||
|
||||
The class provides a method `_stream_response` that can be used to get the response token by token (i.e. word by word). It yields individual tokens from the response.
|
||||
|
||||
Usage:
|
||||
```python
|
||||
for token in _stream_response(response):
|
||||
print(token)
|
||||
```
|
||||
|
@ -1,78 +0,0 @@
|
||||
# WorkerClass Documentation
|
||||
|
||||
## Overview
|
||||
|
||||
The Worker class represents an autonomous agent that can perform tasks through function calls or by running a chat. It can be used to create applications that demand effective user interactions like search engines, human-like conversational bots, or digital assistants.
|
||||
|
||||
The `Worker` class is part of the `swarms.agents` codebase. This module is largely used in Natural Language Processing (NLP) projects where the agent undertakes conversations and other language-specific operations.
|
||||
|
||||
## Class Definition
|
||||
|
||||
The class `Worker` has the following arguments:
|
||||
|
||||
| Argument | Type | Default Value | Description |
|
||||
|-----------------------|---------------|----------------------------------|----------------------------------------------------|
|
||||
| name | str | "Worker" | Name of the agent. |
|
||||
| role | str | "Worker in a swarm" | Role of the agent. |
|
||||
| external_tools | list | None | List of external tools available to the agent. |
|
||||
| human_in_the_loop | bool | False | Determines whether human interaction is required. |
|
||||
| temperature | float | 0.5 | Temperature for the autonomous agent. |
|
||||
| llm | None | None | Language model. |
|
||||
| openai_api_key | str | None | OpenAI API key. |
|
||||
| tools | List[Any] | None | List of tools available to the agent. |
|
||||
| embedding_size | int | 1536 | Size of the word embeddings. |
|
||||
| search_kwargs | dict | {"k": 8} | Search parameters. |
|
||||
| args | Multiple | | Additional arguments that can be passed. |
|
||||
| kwargs | Multiple | | Additional keyword arguments that can be passed. |
|
||||
## Usage
|
||||
|
||||
#### Example 1: Creating and Running an Agent
|
||||
|
||||
```python
|
||||
from swarms import Worker
|
||||
|
||||
worker = Worker(
|
||||
name="My Worker",
|
||||
role="Worker",
|
||||
external_tools=[MyTool1(), MyTool2()],
|
||||
human_in_the_loop=False,
|
||||
temperature=0.5,
|
||||
llm=some_language_model,
|
||||
openai_api_key="my_key",
|
||||
)
|
||||
worker.run("What's the weather in Miami?")
|
||||
```
|
||||
|
||||
#### Example 2: Receiving and Sending Messages
|
||||
|
||||
```python
|
||||
worker.receieve("User", "Hello there!")
|
||||
worker.receieve("User", "Can you tell me something about history?")
|
||||
worker.send()
|
||||
```
|
||||
|
||||
#### Example 3: Setting up Tools
|
||||
|
||||
```python
|
||||
external_tools = [MyTool1(), MyTool2()]
|
||||
worker = Worker(
|
||||
name="My Worker",
|
||||
role="Worker",
|
||||
external_tools=external_tools,
|
||||
human_in_the_loop=False,
|
||||
temperature=0.5,
|
||||
)
|
||||
```
|
||||
|
||||
## Additional Information and Tips
|
||||
|
||||
- The class allows the setting up of tools for the worker to operate effectively. It provides setup facilities for essential computing infrastructure, such as the agent's memory and language model.
|
||||
- By setting the `human_in_the_loop` parameter to True, interactions with the worker can be made more user-centric.
|
||||
- The `openai_api_key` argument can be provided for leveraging the OpenAI infrastructure and services.
|
||||
- A qualified language model can be passed as an instance of the `llm` object, which can be useful when integrating with state-of-the-art text generation engines.
|
||||
|
||||
## References and Resources
|
||||
|
||||
- [OpenAI APIs](https://openai.com)
|
||||
- [Models and Languages at HuggingFace](https://huggingface.co/models)
|
||||
- [Deep Learning and Language Modeling at the Allen Institute for AI](https://allenai.org)
|
@ -1,178 +0,0 @@
|
||||
### Enterprise Grade Documentation
|
||||
|
||||
---
|
||||
|
||||
## AutoScaler Class from `swarms` Package
|
||||
|
||||
The `AutoScaler` class, part of the `swarms` package, provides a dynamic mechanism to handle agents depending on the workload. This document outlines how to use it, complete with import statements and examples.
|
||||
|
||||
---
|
||||
|
||||
### Importing the AutoScaler Class
|
||||
|
||||
Before you can use the `AutoScaler` class, you must import it from the `swarms` package:
|
||||
|
||||
```python
|
||||
from swarms import AutoScaler
|
||||
```
|
||||
|
||||
---
|
||||
|
||||
### Constructor: `AutoScaler.__init__()`
|
||||
|
||||
**Description**:
|
||||
Initializes the `AutoScaler` with a predefined number of agents and sets up configurations for scaling.
|
||||
|
||||
**Parameters**:
|
||||
- `initial_agents (int)`: Initial number of agents. Default is 10.
|
||||
- `scale_up_factor (int)`: Multiplicative factor to scale up the number of agents. Default is 2.
|
||||
- `idle_threshold (float)`: Threshold below which agents are considered idle. Expressed as a ratio (0-1). Default is 0.2.
|
||||
- `busy_threshold (float)`: Threshold above which agents are considered busy. Expressed as a ratio (0-1). Default is 0.7.
|
||||
|
||||
**Returns**:
|
||||
- None
|
||||
|
||||
**Example Usage**:
|
||||
```python
|
||||
from swarms import AutoScaler
|
||||
|
||||
scaler = AutoScaler(
|
||||
initial_agents=5, scale_up_factor=3, idle_threshold=0.1, busy_threshold=0.8
|
||||
)
|
||||
```
|
||||
|
||||
---
|
||||
|
||||
### Method: `AutoScaler.add_task(task)`
|
||||
|
||||
**Description**:
|
||||
Enqueues the specified task into the task queue.
|
||||
|
||||
**Parameters**:
|
||||
- `task`: The task to be added to the queue.
|
||||
|
||||
**Returns**:
|
||||
- None
|
||||
|
||||
**Example Usage**:
|
||||
```python
|
||||
task_data = "Process dataset X"
|
||||
scaler.add_task(task_data)
|
||||
```
|
||||
|
||||
---
|
||||
|
||||
### Method: `AutoScaler.scale_up()`
|
||||
|
||||
**Description**:
|
||||
Scales up the number of agents based on the specified scale-up factor.
|
||||
|
||||
**Parameters**:
|
||||
- None
|
||||
|
||||
**Returns**:
|
||||
- None
|
||||
|
||||
**Example Usage**:
|
||||
```python
|
||||
# Called internally but can be manually invoked if necessary
|
||||
scaler.scale_up()
|
||||
```
|
||||
|
||||
---
|
||||
|
||||
### Method: `AutoScaler.scale_down()`
|
||||
|
||||
**Description**:
|
||||
Scales down the number of agents, ensuring a minimum is always present.
|
||||
|
||||
**Parameters**:
|
||||
- None
|
||||
|
||||
**Returns**:
|
||||
- None
|
||||
|
||||
**Example Usage**:
|
||||
```python
|
||||
# Called internally but can be manually invoked if necessary
|
||||
scaler.scale_down()
|
||||
```
|
||||
|
||||
---
|
||||
|
||||
### Method: `AutoScaler.monitor_and_scale()`
|
||||
|
||||
**Description**:
|
||||
Continuously monitors the task queue and agent utilization to decide on scaling.
|
||||
|
||||
**Parameters**:
|
||||
- None
|
||||
|
||||
**Returns**:
|
||||
- None
|
||||
|
||||
**Example Usage**:
|
||||
```python
|
||||
# This method is internally used as a thread and does not require manual invocation in most scenarios.
|
||||
```
|
||||
|
||||
---
|
||||
|
||||
### Method: `AutoScaler.start()`
|
||||
|
||||
**Description**:
|
||||
Initiates the monitoring process and starts processing tasks from the queue.
|
||||
|
||||
**Parameters**:
|
||||
- None
|
||||
|
||||
**Returns**:
|
||||
- None
|
||||
|
||||
**Example Usage**:
|
||||
```python
|
||||
scaler.start()
|
||||
```
|
||||
|
||||
---
|
||||
|
||||
### Full Usage
|
||||
|
||||
```python
|
||||
from swarms import AutoScaler
|
||||
|
||||
# Initialize the scaler
|
||||
auto_scaler = AutoScaler(
|
||||
initial_agents=15, scale_up_factor=2, idle_threshold=0.2, busy_threshold=0.7
|
||||
)
|
||||
|
||||
# Start the monitoring and task processing
|
||||
auto_scaler.start()
|
||||
|
||||
# Simulate the addition of tasks
|
||||
for i in range(100):
|
||||
auto_scaler.add_task(f"Task {i}")
|
||||
```
|
||||
|
||||
### Pass in Custom Agent
|
||||
You can pass any agent class that adheres to the required interface (like having a run() method). If no class is passed, it defaults to using AutoBot. This makes the AutoScaler more flexible and able to handle a wider range of agent implementations.
|
||||
|
||||
```python
|
||||
from swarms import AutoScaler
|
||||
|
||||
auto_scaler = AutoScaler(agent=YourCustomAgent)
|
||||
auto_scaler.start()
|
||||
|
||||
for i in range(100): # Adding tasks
|
||||
auto_scaler.add_task(f"Task {i}")
|
||||
```
|
||||
|
||||
|
||||
---
|
||||
|
||||
**Notes**:
|
||||
1. Adjust the thresholds and scaling factors as per your specific requirements and nature of the tasks.
|
||||
2. The provided implementation is a baseline. Depending on your production environment, you may need additional features, error-handling, and optimizations.
|
||||
3. Ensure that the `swarms` package and its dependencies are installed in your environment.
|
||||
|
||||
---
|
@ -1,147 +0,0 @@
|
||||
# Module Name: Group Chat
|
||||
|
||||
The `GroupChat` class is used to create a group chat containing a list of agents. This class is used in scenarios such as role-play games or collaborative simulations, where multiple agents must interact with each other. It provides functionalities to select the next speaker, format chat history, reset the chat, and access details of the agents.
|
||||
|
||||
## Class Definition
|
||||
|
||||
The `GroupChat` class is defined as follows:
|
||||
|
||||
```python
|
||||
@dataclass
|
||||
class GroupChat:
|
||||
"""
|
||||
A group chat class that contains a list of agents and the maximum number of rounds.
|
||||
|
||||
Args:
|
||||
agents: List[Agent]
|
||||
messages: List[Dict]
|
||||
max_round: int
|
||||
admin_name: str
|
||||
|
||||
Usage:
|
||||
>>> from swarms import GroupChat
|
||||
>>> from swarms.structs.agent import Agent
|
||||
>>> agents = Agent()
|
||||
"""
|
||||
|
||||
agents: List[Agent]
|
||||
messages: List[Dict]
|
||||
max_round: int = 10
|
||||
admin_name: str = "Admin" # the name of the admin agent
|
||||
```
|
||||
|
||||
## Arguments
|
||||
|
||||
The `GroupChat` class takes the following arguments:
|
||||
| Argument | Type | Description | Default Value |
|
||||
|-------------|---------------|---------------------------------------------------|-----------------|
|
||||
| agents | List[Agent] | List of agents participating in the group chat. | |
|
||||
| messages | List[Dict] | List of messages exchanged in the group chat. | |
|
||||
| max_round | int | Maximum number of rounds for the group chat. | 10 |
|
||||
| admin_name | str | Name of the admin agent. | "Admin" |
|
||||
|
||||
## Methods
|
||||
|
||||
1. **agent_names**
|
||||
- Returns the names of the agents in the group chat.
|
||||
- Returns: List of strings.
|
||||
|
||||
2. **reset**
|
||||
- Resets the group chat, clears all the messages.
|
||||
|
||||
3. **agent_by_name**
|
||||
- Finds an agent in the group chat by their name.
|
||||
- Arguments: name (str) - Name of the agent to search for.
|
||||
- Returns: Agent - The agent with the matching name.
|
||||
- Raises: ValueError if no matching agent is found.
|
||||
|
||||
4. **next_agent**
|
||||
- Returns the next agent in the list based on the order of agents.
|
||||
- Arguments: agent (Agent) - The current agent.
|
||||
- Returns: Agent - The next agent in the list.
|
||||
|
||||
5. **select_speaker_msg**
|
||||
- Returns the message for selecting the next speaker.
|
||||
|
||||
6. **select_speaker**
|
||||
- Selects the next speaker based on the system message and history of conversations.
|
||||
- Arguments: last_speaker (Agent) - The speaker in the last round, selector (Agent) - The agent responsible for selecting the next speaker.
|
||||
- Returns: Agent - The agent selected as the next speaker.
|
||||
|
||||
7. **_participant_roles**
|
||||
- Formats and returns a string containing the roles of the participants.
|
||||
- (Internal method, not intended for direct usage)
|
||||
|
||||
8. **format_history**
|
||||
- Formats the history of messages exchanged in the group chat.
|
||||
- Arguments: messages (List[Dict]) - List of messages.
|
||||
- Returns: str - Formatted history of messages.
|
||||
|
||||
## Additional Information
|
||||
|
||||
- For operations involving roles and conversations, the system messages and agent names are used.
|
||||
- The `select_speaker` method warns when the number of agents is less than 3, indicating that direct communication might be more efficient.
|
||||
|
||||
## Usage Example 1
|
||||
|
||||
```Python
|
||||
from swarms import GroupChat
|
||||
from swarms.structs.agent import Agent
|
||||
|
||||
agents = [Agent(name="Alice"), Agent(name="Bob"), Agent(name="Charlie")]
|
||||
group_chat = GroupChat(agents, [], max_round=5)
|
||||
|
||||
print(group_chat.agent_names) # Output: ["Alice", "Bob", "Charlie"]
|
||||
|
||||
selector = agents[1]
|
||||
next_speaker = group_chat.select_speaker(last_speaker=agents[0], selector=selector)
|
||||
print(next_speaker.name) # Output: "Bob"
|
||||
```
|
||||
|
||||
## Usage Example 2
|
||||
|
||||
```Python
|
||||
from swarms import GroupChat
|
||||
from swarms.structs.agent import Agent
|
||||
|
||||
agents = [Agent(name="X"), Agent(name="Y")]
|
||||
group_chat = GroupChat(agents, [], max_round=10)
|
||||
|
||||
group_chat.messages.append({"role": "X", "content": "Hello Y!"})
|
||||
group_chat.messages.append({"role": "Y", "content": "Hi X!"})
|
||||
|
||||
formatted_history = group_chat.format_history(group_chat.messages)
|
||||
print(formatted_history)
|
||||
"""
|
||||
Output:
|
||||
'X: Hello Y!
|
||||
Y: Hi X!'
|
||||
"""
|
||||
|
||||
agent_charlie = Agent(name="Charlie")
|
||||
group_chat.agents.append(agent_charlie)
|
||||
|
||||
print(group_chat.agent_names) # Output: ["X", "Y", "Charlie"]
|
||||
```
|
||||
|
||||
## Usage Example 3
|
||||
|
||||
```Python
|
||||
from swarms import GroupChat
|
||||
from swarms.structs.agent import Agent
|
||||
|
||||
agents = [Agent(name="A1"), Agent(name="A2"), Agent(name="A3")]
|
||||
group_chat = GroupChat(agents, [], max_round=3, admin_name="A1")
|
||||
|
||||
group_chat.reset()
|
||||
print(group_chat.messages) # Output: []
|
||||
```
|
||||
|
||||
## References
|
||||
|
||||
1. [Swarms Documentation](https://docs.swarms.org/)
|
||||
2. [Role-Based Conversations in Multi-Agent Systems](https://arxiv.org/abs/2010.01539)
|
||||
|
||||
This detailed documentation has provided a comprehensive understanding of the `GroupChat` class in the `swarms.structs` module of the `swarms` library. It includes class definition, method descriptions, argument types, and usage examples.
|
||||
|
||||
*(Sample Documentation - 950 words)*
|
Loading…
Reference in new issue